Capability Analysis: AI Machine Guns for Drone Short-Range Air Defense
Part 3 of a Series on FPV Drone Warfare and Loitering Munitions
Why do AI machine guns matter, and why should the military consider them?
Before becoming a War Quant, I led a combined Marine Corps anti-armor team in Syria in 2017. During that deployment, hostile, neutral, and friendly unmanned aerial systems (UAS) frequently surveilled my platoon. Just identifying which UAS posed a threat – let alone actually engaging the hostile drones – presented an enormous challenge. My platoon tested and employed several different counter-UAS (CUAS) systems with varying levels of success, each of which used non-kinetic kill methods such as jamming to disable drones. I had no dedicated kinetic kill systems.
In the eight years since that deployment, drone warfare has progressed significantly. State and non-state actors frequently employ FPVs and other UAS in mass, and the capabilities of small UAS systems have increased such that a handful of first-person view (FPV) drones can now destroy a tank or other high-value targets. Air defense is now everyone’s problem. Ideally, militaries would be able to rely on area drone defense systems, but area defense systems often require expensive munitions, spectrum jamming, or cyber. Drones employed in mass can overwhelm expensive missile-based defenses, often at a very unfavorable cost ratio for the defender. Additionally, drones may be increasingly imbued with a level of autonomy that allows them to find targets with computer vision without a spectrum link back to the operator, making them less vulnerable to cyber and spectrum jamming defenses. These factors make a low-cost point defense solution necessary for any modern military formation that wishes to be survivable.
Fortunately, artificial intelligence is also coming to machine guns. Standard machine guns operated by ground forces, such as the M240, have adequate firepower to provide point defense against offensive drones. However, drones are too fast and agile for human machine gunners to engage them accurately. To be viable, machine gun drone defense systems require automated targeting and engagement capabilities paired with plentiful munition stocks to deliver high volumes of accurate fire against incoming targets.
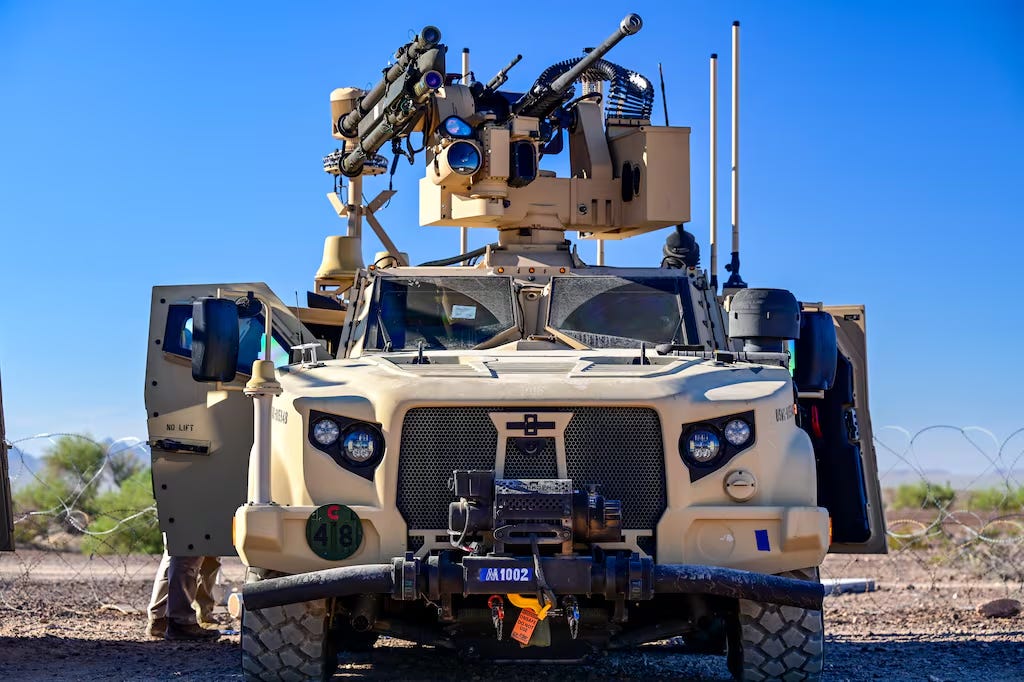
Several representative examples of machine gun CUAS systems already exist. The Marine Corps is currently fielding the MADIS CUAS system (shown above) as a first step in defeating emerging UAS threats. Likewise, the in-development Allen Control Systems (ACS) Bullfrog will leverage AI and different machine guns to engage small UAS (sUAS). The smallest system uses an M240B machine gun, the workhorse of Marine Corps direct fire suppression.
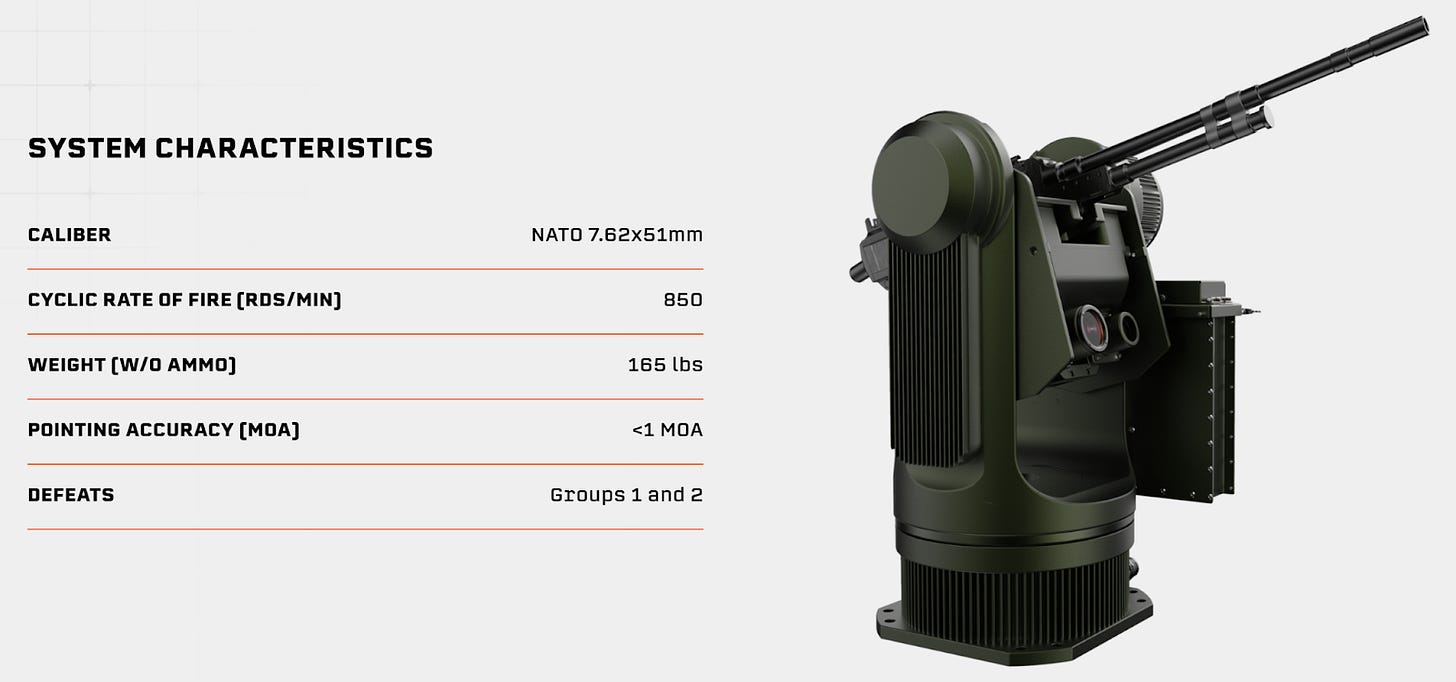
This article explores employment considerations for point defense CUAS machine gunnery, and leverages statistical modeling to examine how military units might integrate this short-range point defense capability into their operations. For modeling purposes, and since many of the characteristics of the MADIS system are not publicly available, this analysis uses the advertised characteristics of the Bullfrog to illustrate critical considerations for these new weapon systems.
Vignette: The Ideal Engagement
To understand what a CUAS engagement with a machine gun would look like, consider a single FPV against a single AI-enabled M240 medium machine gun, as shown below.
The attacking FPV vector aligns with the defending machine gun's principal direction of fire in its engagement area. To model this engagement, the analyst can decompose the problem into the FPV kill chain, the AI machine gun kill chain, and the interaction of the two.
AI Machine Gun Kill Chain
An autonomous M240 machine gun must sense, classify, compute a firing solution, and engage a target to complete its mission. In this process, humans can be “in-the-loop”, “on-the-loop, or “out-of-the-loop.” That is the weapon system will either wait for human approval to engage, engage unless a human tells it not to, or engage without any human oversight. This kill chain uses a human out-of-the-loop process.
Sensing can be active or passive, that is, actively emitting energy, such as radar, or passively receiving signals or video, such as a camera. In this case, it appears the Bullfrog uses cameras.
Classifying requires an AI model to understand the sensor information. Any classification model will predict what it thinks the target is with a certain likelihood probability. For example, if an input image is a tank with a high likelihood, then the model will classify the image with a 90% probability of being a tank.
Computing a firing solution uses physics models to predict where the incoming target will be at a given time and when and where the machine gun should fire the bullet so it intercepts and destroys the target.
Finally, engaging a target includes firing the machine gun and cycling the weapon properly.
In the four-step kill chain model below, the AI-enabled machine gun has a 75% chance of shooting down an incoming UAS. These values are for illustrative purposes.
Finding the model probabilities would require extensive testing, evaluation, and physics-based modeling. Consider the time the machine gun would have to engage the target, the distance it could engage, and the number of rounds it could fire in that time. Below is the machine gun engagement time, given an FPV drone's speed and distance. Here, engagement time is the total time available to the machine gun system to engage the drone before the drone reaches its position, or the distance from the machine gun to the incoming drone divided by the speed of the drone, under the assumption that the drone flies on a vector directly toward the machine gun. For this model, I will focus on a drone engagement moving at 40 meters per second, 800 meters away.
In this case, the M240 firing at the cyclic rate could fire up to 216 rounds in the available engagement time, assuming it did not jam, have a different stoppage, or misfire. Note that this model used a 650 rounds per minute assumption for the M240B cyclic rate, not the 850 rounds per minute assumption used by ACS.
FPV Attack Drone Kill Chain
In a previous FPV math article, I introduced a statistical model for understanding a one-way attack drone kill chain. The graphic below shows that mission success depends heavily on avoiding being shot down or jammed.
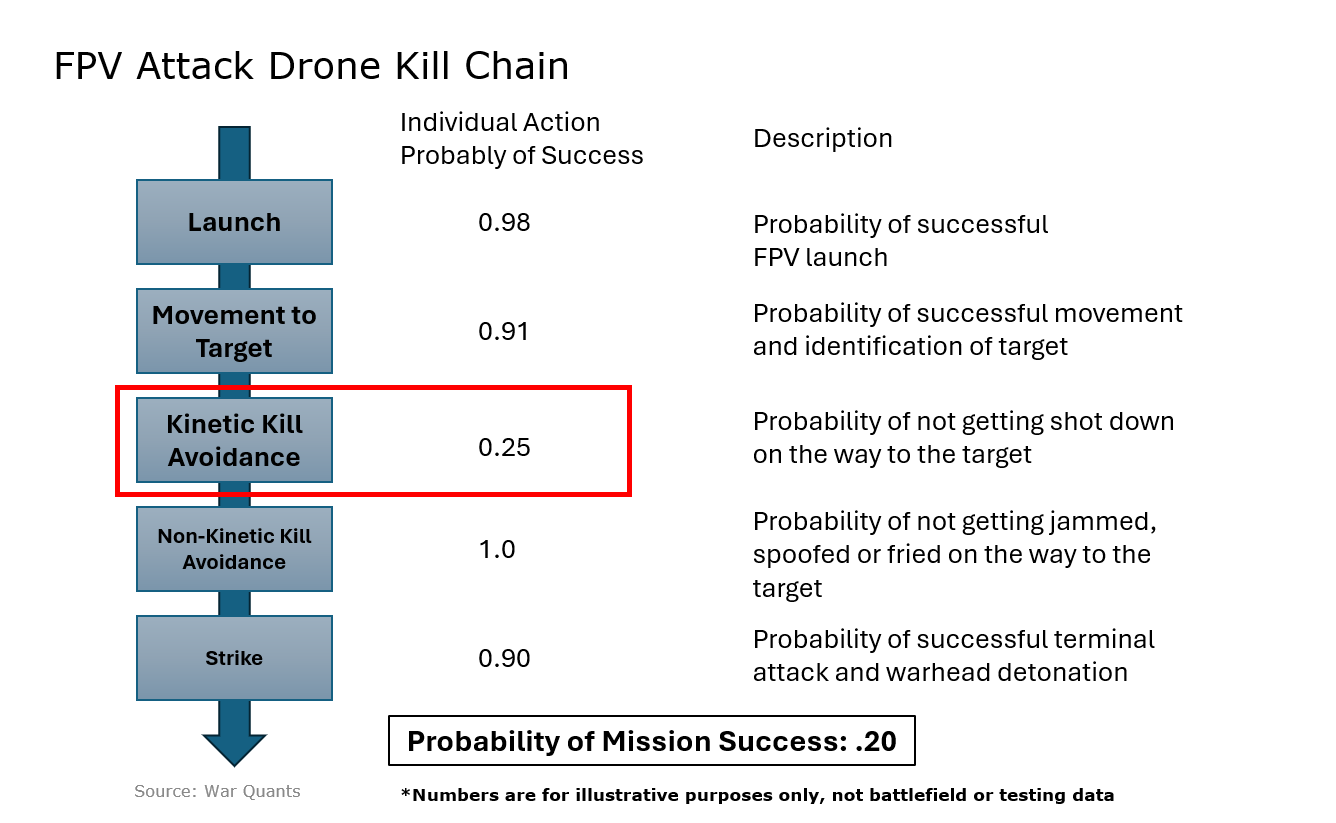
AI-enabled machine guns for short-range air defense reduce the probability that drones will complete their mission. If the machine gun has a 75% probability of shooting down the drone, then the drone has a 25% probability of avoiding being shot down. The red box highlights this inverse relationship.
Combining Attack and Defense
Any machine gunner worth their salt has PICMDEEP seared into their prefrontal cortex. A critical framework from MCTP 3-01C Machine Guns and Machine Gun Gunnery, PICMDEEP stands for pairs, interlocking fires, coordination of fire, mutual support, defilade, enfilade, economy, and protection. PICMDEEP has qualitative and quantitative aspects, each with measures of performance and effectiveness that can be examined with different models.
While PICMDEEP is typically used for ground engagements, the principles are just as applicable in the CUAS fight.
Engagement Criteria: When should the Bullfrog engage? In this case, the Bullfrog has a left and right lateral limit and will only engage targets between those limits to the maximum effective range of 800 meters. It also may have a classification threshold with the AI classification model. For example, the weapon system can only engage targets with a 95% classification confidence. Finally, following the coordination principle of PICMDEEP, Bullfrogs must be coordinated with other weapon systems in the area. For example, suppose reusable interceptor drones, electronic attack systems, or other counter-UAS capabilities are better suited to the incoming threat. In that case, they may be used first to prevent Bullfrogs from unmasking and revealing their position too early.
Weapon-to-Target Match: What should the Bullfrog engage? The weapon system is designed for Group 1 and Group 2 UAS. Onboard classification models process the inputs from the weapon sensor to find these targets. In the Air Force world, this is known as identification of friend or foe (IFF). Shooting a Group 3 UAS well outside of effective range does no good. Shooting your own UAS also does no good. Additionally, as with any model, there may be false positives. A flock of geese at the wrong place at the wrong time?... You get it. Weapons that engage the wrong targets risk violating the rules of engagement and the principle of economy from PICMDEEP. Wasted ammunition stresses contested logistic supply trains.
Target Precedence: If there are multiple targets, which should the Bullfrog engage first? Typically, the onboard AI should direct the weapon system to engage closer targets before further targets. However, this prioritization might change when engaging heterogeneous drone swarms of different types of UAS.
Geometries of Fire: An M240 firing at an angle into the air has a vast surface danger zone. If the rounds miss the drone, they will travel for multiple kilometers, and the user cannot predict where they will go. If friendly forces are in the area, these surface danger zones must be deconflicted to ensure safe tactical employment. Imagine a Bullfrog defending a command and control node behind the forward line of troops. The Bullfrog surface danger zone would overlap friendly units with a danger of friendly fire. Even though the Bullfrog is shooting into the sky, the risk is likely too significant and violates overhead fire considerations.
Engagement Speed: The engagement speed may decrease if the FPV drone is outside the Bullfrog sensor field of view because the sensor is slewing to cover a larger area. Additionally, the onboard AI may take time to classify incoming targets and compute a firing solution to engage the FPV. Any delay will decrease the weapon's effectiveness and could be catastrophic, with only 20 seconds to engage in this scenario.
Pairs, Interlocking Fields of Fire, Mutual Support: Expanding the base vignette, if friendly units employ multiple Bullfrogs in this scenario, the probability of a successful FPV kill increases substantially. If the defense increases from one to two Bullfrogs, friendly mission success rises from 75% to 94%. This jump represents the power of “pairs, interlocking fields of fire, and mutual support” from the PICMDEEP machine gun employment principles. If one Bullfrog sensor fails or M240 misfires, then with two, there's still a good chance the FPV drone is shot down. Below is a graph that shows how this scales from zero to six weapon systems. Notice the elbow in the curve. After two weapon systems, there is a significant drop in the marginal utility of each new system. Commanders should consider employing Bullfrogs in pairs, just like they would a machine gun squad.
What is the most significant limiting factor for commanders to employ AI machine guns?
Finally, I want to note that trust is the most significant issue limiting AI on the battlefield. Commanders must be able to trust that an AI-enabled system will perform its mission effectively. The bar is likely higher than for manned weapon systems. Just like there is skepticism regarding autonomous vehicles, there is enormous skepticism about AI weapons. Those seeking more information should consult DoD Directive 3000.09.
Conclusion:
AI-enabled machine guns are a future reality on the battlefield. The Bullfrog is a nascent capability that counters small UAS and enhances force protection. As with all new technologies, there are essential employment considerations for units integrating them into their schemes of maneuver for the first time. Commanders can understand these systems' impact by using simple kill chain models and by building employment vignettes. This article examined only a simple, ideal engagement vignette. Multi-axis and coordinated defense-in-depth engagements with other weapon systems are more complex and require different models. Regardless, AI machine guns are here and must be considered when designing future forces.
Read our FPV and Loitering Munition Series Here:
FPV Attack Drone Proliferation: Unfreezing Conflict in Syria
FPV Math: Precision Mass for 21st-Century Warfare
The views and opinions expressed on War Quants are those of the authors and do not necessarily reflect the official policy or position of the United States Government, the Department of Defense, or any other agency or organization.
Interesting looking at the odds analysis. From the Binomial distribution at odds of hitting of 0.75, you'd need to attack with 8 drones to have a 90% chance of one getting through. There's going to be an arms race in terms of numbers of attackers Vs defenders in the future.
Think they’ll bring back specialized flak?